Data Quality Dimensions help us measure and monitor the quality of our data. Data quality issues are easy to recognize, but difficult to measure. Furthermore, companies can observe the profound effect of Data Quality on business operations, decision-making, and customer satisfaction. However, many companies still do not systematically measure their Data Quality. It is imperative for organizations to move from a reactive stance on Data Quality to a proactive stance.
Companies rely on six data quality dimensions to gain a better picture of their company’s data quality. In this article, we’ll dive into these dimensions and explain how they apply in various situations.
Data Quality Dimensions: Table of Contents
Six Data Quality Dimensions to Measure and Monitor
1. Accuracy
Data accuracy describes how consistently correct an organization’s data is. Data Accuracy metrics provides information on how closely data reflects actual values and confirms whether it comes from a verifiable source. Accuracy can be difficult to measure for data points that don’t have a definitive source of truth.
Highly accurate data allows companies to base their business decisions on reliable information. Accuracy is especially crucial in industries with a strict regulatory environment, such as healthcare and finance. For instance, inaccurate data can lead to doctors misdiagnosing patients and giving them incorrect treatments. In finance, inaccurate reporting can expose companies to regulatory penalties and financial loss.
2. Completeness
Data completeness measures whether a dataset contains all the expected data elements. Furthermore, completeness metrics ensure all pertinent information needed is present and that the data is fit for use, whatever that may be.
Complete data often means all necessary fields and values are available for analysis. Otherwise, it won’t be as useful or can even disrupt business operations. In an industrial setting a lack of complete data can lead to inefficient production processes. If an employee fails to fill in machine allocations for each production run, the machine will either sit idle or be scheduled for multiple jobs at once. Both options require manual intervention and compromise productivity.
3. Consistency
Data consistency refers to the uniformity of data across various applications, databases, and systems. Regardless of the platform, data needs to maintain its format, standard, and value throughout an organizations data ecosystem. This isn’t limited to individual data points, but also aggregates.
In the retail industry consistency of product information is critical. Product descriptions, prices, and SKU numbers need to remain the same across online stores, physical stores, and internal databases. For instance, t-shirts should have the same description, size options, price, and SKU in e-commerce, in physical stores, and in inventory management systems. Inconsistencies can confuse customers or cause operational issues such as selling an item at the wrong price.
4. Uniqueness
Data uniqueness is the data quality dimension responsible for keeping out duplicates or redundancy. It identifies cases where a record has multiple data entries.
Uniqueness is important because duplicate data leads to a host of problems across industries. If an insurance company has duplicate records for the same policyholder, that will lead to inconsistencies in the way that customer is treated. Poor customer experience in any industry leads to churn.
5. Timeliness
Data timeliness ensures companies have relevant insights on hand when users need them. An up-to-date data set provides timely decision-making and management support because it represents reality at the time the information is needed.
This data quality dimension is considered related to data accuracy as accuracy decays over time. For instance, insurance companies can provide new customers incorrect policy data if the providers are using outdated risk data for as reference.
6. Data Validity
Validity of data means that data conforms to defined business rules and constraints, ensuring its relevance and correctness. Data is also considered valid when it matches its definition’s syntax (format, type, range).
This data quality dimension comes into play when a customer has been allowed to enter an invalid shipping address due to a lack of address validation. Products will be shipped to an address that does not exist and have to be reshipped, resulting in increased costs for the business and poor customer experience.
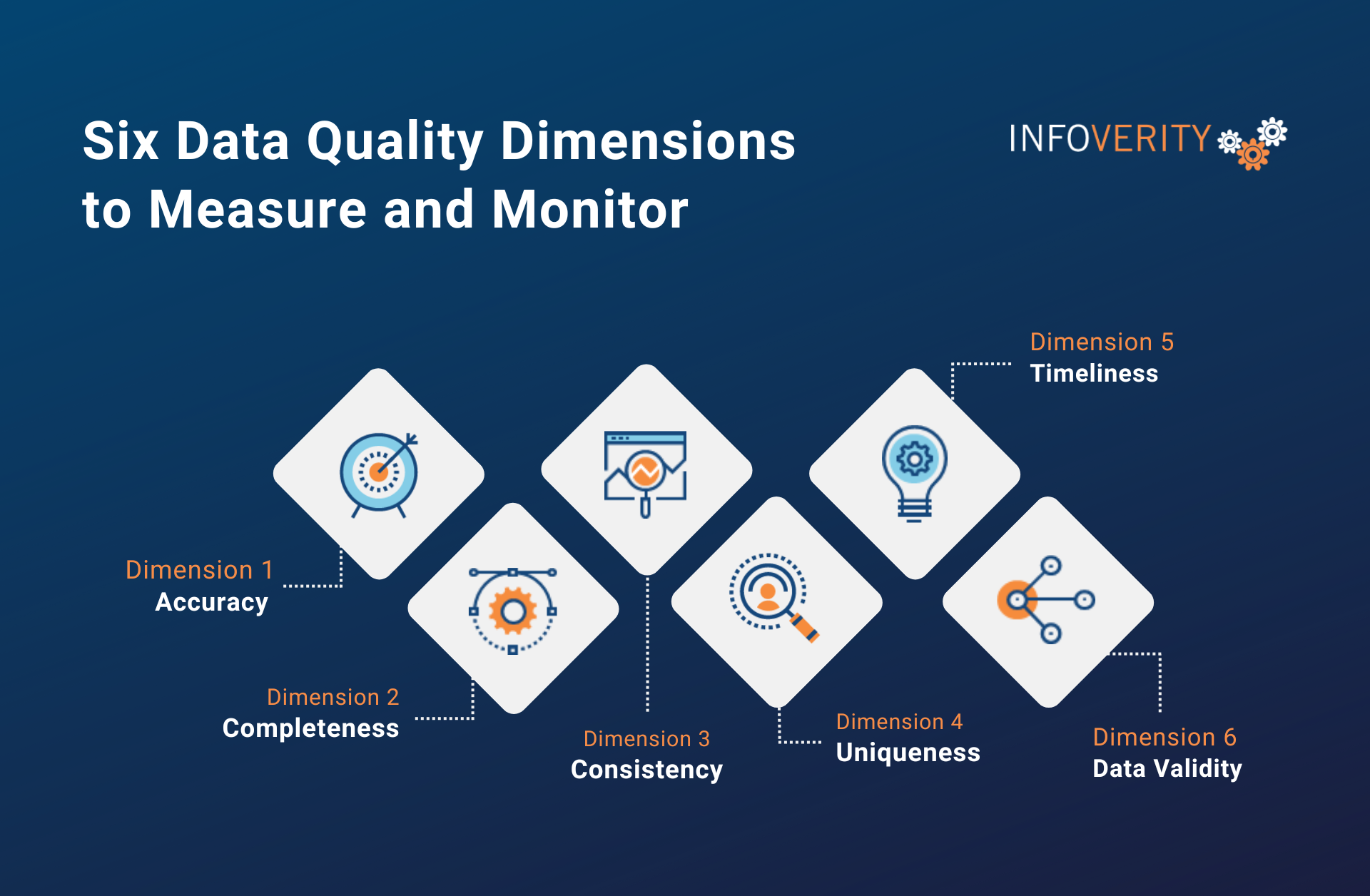
The Impact of Data Quality on Operations and Decision-Making
High data quality contributes to business efficiency. It also equips organizations with better insights for making informed strategic decisions.
For instance, manufacturers with high-quality data can accurately track inventory levels, streamline supply chain operations, and reduce waste, leading to more efficient production processes. Analyzing production metrics can help them forecast demand accurately and adjust production schedules to maximize productivity and profitability.
Poor data quality, on the other hand, can significantly harm business operations. Inaccurate or incomplete data can lead to operational failures, such as mismanagement of inventory or inefficient processes.
This is why Infoverity recommends that organizations put processes in place to automatically monitor the six dimensions of data quality. Data Quality monitoring is a critical aspect of any organization’s data strategy. And the first step to enabling proactive Data Quality management.
The Role of Process, People, and Technology in Improving and Maintaining High Data Quality
Without ongoing maintenance, a one-time data quality fix might resolve existing data issues temporarily, but errors, duplicates, and inconsistencies will quickly rear their ugly head again.
That’s because data quality management isn’t one and done. It involves recurring processes that identify and fix inconsistencies, errors, and inaccuracies. In addition, maintaining high data standards requires regular data audits, continuous monitoring for data quality issues, and real-time validation checks to track and monitor data quality dimensions.
Organizations looking to improve data quality often invest in data quality tools to resolve their problems. However, a data quality tool alone is not enough to move the needle on Data Quality. Data quality requires that members of the business engage in consistent processes to improve and maintain the quality of data. On the other hand, data governance is necessary to establish the foundation for enterprise-scale data quality.
What about data ownership?
Companies need to develop a strong framework to enforce policies and establish data ownership. That is, a comprehensive framework for managing, securing, and using data effectively enables organizations to cultivate a data driven culture. IT departments can take care of technical data management, while it is every employee’s responsibility to keep quality in check. Training staff on the value of data quality practices and the importance of accurate data should be part of the company’s initiatives.
With data governance and data quality integrated into data management tools, offering features like profiling, automated data validation, and data cleansing software, organizations are more capable than ever when taking the reigns on data quality. Also, additional tools such as master data management (MDM) systems can automate the improvement of data quality and create trust in data. With the latest data quality tools available, organizations can automate the process of identifying and correcting data anomalies by utilizing machine learning algorithms and other advanced analytics techniques.
Maintaining High Data Quality: A Necessity for Successful Business Operations
High-quality data is what allows companies to make informed business decisions in a timely manner. However, maintaining the quality of an organization’s data is hard work. At Infoverity, we understand the challenges and decisions that organizations face as they navigate the path to becoming a data-driven organization.
We’re here to support you on this journey. Find out how we can help. Or get in touch with us to learn more about what we can do to transform your organization.
FAQ
What is data quality?
Data quality refers to the accuracy, completeness, consistency, reliability, and timeliness of data, ensuring it is fit for its intended use. High-quality data contributes to business efficiency. It is what equips organizations with better insights and allows them to make informed business decisions in a timely manner.
What are the six dimensions of data quality?
Companies rely on six data quality dimensions to gain a better picture of their company's data quality: accuracy, completeness, consistency, uniqueness, timeliness and data validity.
How to measure data quality dimensions?
Data Quality monitoring is a critical aspect of any organization’s data strategy. Data Quality issues are easy to recognize, but difficult to measure. Companies can observe the profound effect on business operations, decision-making, and customer satisfaction.
How to make data quality decisions?
A comprehensive framework for managing, securing, and using data effectively enables organizations to cultivate a data driven culture. With the latest data quality tools available, organizations can automate the process of identifying and correcting data anomalies by utilizing machine learning algorithms and other advanced analytics techniques.
Download The Guide
Looking for a guide to deploy non-invasive data governance across your organization?
Download our free white paper and discover how to implement data governance seamlessly at your company.